Type de document : article scientifique publié dans Animal
Auteurs : S. Benaissa, F.A.M. Tuyttens, D. Plets, L. Martens, L. Vandaele, W. Joseph, B. Sonck
Résumé en français (traduction) : Amélioration du suivi du comportement des bovins grâce à la combinaison des données de localisation à bande ultra-large et des données d’accélérométrie
Le comportement des bovins est fondamentalement lié à leur santé, (re)production et bien-être. L’objectif de cette étude était de présenter une méthode efficace pour intégrer les données de localisation à bande ultra-large (UWB) en bâtiment et les données d’accéléromètre afin d’améliorer les systèmes de surveillance du comportement des bovins. Au total, 30 vaches laitières ont été équipées de balises de suivi portables UWB Pozyx (Pozyx, Gand, Belgique) sur la partie supérieure (dorsale) du cou de la vache. Outre les données de localisation, l’étiquette Pozyx fournit également des données d’accélérométrie. La combinaison des données des deux capteurs a été réalisée en deux étapes. Dans un premier temps, le temps réel passé dans les différentes zones de l’étable a été calculé à l’aide des données de localisation. Dans un deuxième temps, les données de l’accéléromètre ont été utilisées pour classer le comportement des vaches à l’aide des informations de localisation de l’étape 1 (par exemple, une vache située dans les logettes ne peut pas être classée comme s’alimentant ou buvant). Au total, 156 heures d’enregistrements vidéo ont été utilisées pour la validation. Pour chaque heure de données, le temps total passé par chaque vache dans chaque zone et les comportements qu’elle a adoptés (se nourrir, boire, ruminer, se reposer et manger du concentré) ont été calculés à l’aide des capteurs et comparés aux enregistrements vidéo annotés. Des diagrammes de Bland-Altman pour la corrélation et la différence entre les capteurs et l’enregistrement vidéo ont ensuite été calculés pour l’analyse des performances. La performance globale de localisation des animaux dans les zones fonctionnelles correctes était très élevée. Le R2 était de 0,99 (P ≤ 0,001) et la racine de l’erreur quadratique moyenne (RMSE) était de 1,4 min (7,5 % du temps total). Les meilleures performances ont été obtenues pour les zones d’alimentation et de couchage (R2 = 0,99, P ≤ 0,001). Les performances étaient plus faibles dans l’aire d’abreuvement (R2 = 0,90, P ≤ 0,01) et dans l’aire d’alimentation en concentré (R2 = 0,85, P ≤ 0,05). Pour la combinaison des données de localisation et d’accélérométrie, une performance globale élevée (tous les comportements) a été obtenue avec un R2 de 0,99 (P ≤ 0,001) et une RMSE de 1,6 min (12 % du temps total). La combinaison des données de localisation et d’accélérométrie a amélioré la RMSE du temps d’alimentation et du temps de rumination par rapport aux données d’accélérométrie seules (2,6-1,4 min). En outre, la combinaison de la localisation et de l’accélérométrie a permis une classification précise de comportements supplémentaires difficiles à détecter à l’aide de l’accéléromètre seul, tels que la consommation de concentrés et d’eau (R2 = 0,85 et 0,90, respectivement). Cette étude démontre le potentiel de la combinaison de l’accéléromètre et des données de localisation UWB pour la conception d’un système de surveillance robuste pour les bovins laitiers.
Résumé en anglais (original) : Cattle behaviour is fundamentally linked to the cows’ health, (re)production, and welfare. The aim of this study was to present an efficient method to incorporate Ultra-Wideband (UWB) indoor location and accelerometer data for improved cattle behaviour monitoring systems. In total, 30 dairy cows were fitted with UWB Pozyx wearable tracking tags (Pozyx, Ghent, Belgium) on the upper (dorsal) side of the cow’s neck. In addition to the location data, the Pozyx tag reports accelerometer data as well. The combination of both sensor data was performed in two steps. In the first step, the actual time spent in the different barn areas was calculated using location data. In the second step, accelerometer data were used to classify cow behaviour using the location information of step 1 (e.g., a cow located in the cubicles cannot be classified as feeding, or drinking). A total of 156 hours of video recordings were used for the validation. For each hour of data, the total time each cow spent in each area and performing which behaviours (feeding, drinking, ruminating, resting, and eating concentrates) were computed using the sensors and compared against annotated video recordings. Bland-Altman plots for the correlation and difference between the sensors and the video recording were then computed for the performance analysis. The overall performance of locating the animals into the correct functional areas was very high. The R2 was 0.99 (P ≤ 0.001), and the root-mean-square error (RMSE) was 1.4 min (7.5% of the total time). The best performance was obtained for the feeding and lying areas (R2 = 0.99, P ≤ 0.001). Performance was lower in the drinking area (R2 = 0.90, P ≤ 0.01) and the concentrate feeder (R2 = 0.85, P ≤ 0.05). For the combined location + accelerometer data, high overall performance (all behaviours) was obtained with an R2 of 0.99 (P ≤ 0.001) and a RMSE of 1.6 min (12% of the total time). The combination of location and accelerometer data improved the RMSE of the feeding time and ruminating time compared to the accelerometer data alone (2.6–1.4 min). Moreover, the combination of location and accelerometer enabled accurate classification of additional behaviours that are difficult to detect using the accelerometer alone, such as eating concentrates and drinking (R2 = 0.85 and 0.90, respectively). This study demonstrates the potential of combining accelerometer and UWB location data for the design of a robust monitoring system for dairy cattle.
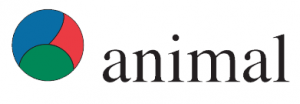